Why do recurrent layers work better than simple feed-forward networks?Character recognition neural net...
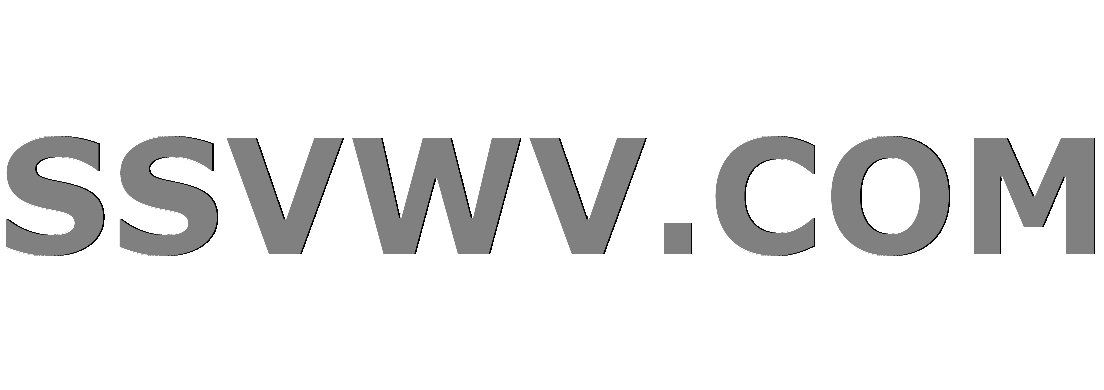
Multi tool use
Why would the Pakistan airspace closure cancel flights not headed to Pakistan itself?
What is the best way to simulate grief?
Integral inequality of length of curve
What's a good word to describe a public place that looks like it wouldn't be rough?
1 0 1 0 1 0 1 0 1 0 1
What happens if a wizard reaches level 20 but has no 3rd-level spells that they can use with the Signature Spells feature?
Strange Sign on Lab Door
Issues with new Macs: Hardware makes them difficult for me to use. What options might be available in the future?
It took me a lot of time to make this, pls like. (YouTube Comments #1)
How should I handle players who ignore the session zero agreement?
How can I deal with a significant flaw I found in my previous supervisor’s paper?
Pendulum Rotation
What formula could mimic the following curve?
Using loops to create tables
How to deal with an incendiary email that was recalled
Dilemma of explaining to interviewer that he is the reason for declining second interview
Number of FLOP (Floating Point Operations) for exponentiation
The vanishing of sum of coefficients: symmetric polynomials
What's the rationale behind the objections to these measures against human trafficking?
Unwarranted claim of higher degree of accuracy in zircon geochronology
Overfitting and Underfitting
Is it a fallacy if someone claims they need an explanation for every word of your argument to the point where they don't understand common terms?
Is there a better way to make this?
How can I introduce myself to a party without saying that I am a rogue?
Why do recurrent layers work better than simple feed-forward networks?
Character recognition neural net topology/designWhy is vanishing gradient a problem?SGD learning gets stuck when using a max pooling layer (but it works fine with just conv + fc)Feeding back hidden state manually in tf.nn.dynamic_rnn (Tensorflow)Training an RNN with examples of different lengths in KerasWhat principle is behind semantic segmenation with CNNs?1d time series to time series approximation using deep learningUnderstanding Timestamps and Batchsize of Keras LSTM considering Hiddenstates and TBPTTUnderstanding LSTM structure1x1 convolutions, equivalence with fully connected layer
$begingroup$
On a time series problem that we try to solve using RNNs
, the input usually has the shape $input features times timesteps times batchsize$ and we then feed this input into recurrent layers. An alternative would be to flatten the data so that the shape is $(input features times timesteps) times batchsize$ and use a fully connected layer for our time series task. This would clearly work and our dense network
would be able to find dependencies between the data at different timesteps as well. So what is it that makes recurrent layers more powerful? I would be very thankful for an intuitive explanation.
machine-learning neural-network deep-learning lstm rnn
New contributor
Zubera is a new contributor to this site. Take care in asking for clarification, commenting, and answering.
Check out our Code of Conduct.
$endgroup$
add a comment |
$begingroup$
On a time series problem that we try to solve using RNNs
, the input usually has the shape $input features times timesteps times batchsize$ and we then feed this input into recurrent layers. An alternative would be to flatten the data so that the shape is $(input features times timesteps) times batchsize$ and use a fully connected layer for our time series task. This would clearly work and our dense network
would be able to find dependencies between the data at different timesteps as well. So what is it that makes recurrent layers more powerful? I would be very thankful for an intuitive explanation.
machine-learning neural-network deep-learning lstm rnn
New contributor
Zubera is a new contributor to this site. Take care in asking for clarification, commenting, and answering.
Check out our Code of Conduct.
$endgroup$
add a comment |
$begingroup$
On a time series problem that we try to solve using RNNs
, the input usually has the shape $input features times timesteps times batchsize$ and we then feed this input into recurrent layers. An alternative would be to flatten the data so that the shape is $(input features times timesteps) times batchsize$ and use a fully connected layer for our time series task. This would clearly work and our dense network
would be able to find dependencies between the data at different timesteps as well. So what is it that makes recurrent layers more powerful? I would be very thankful for an intuitive explanation.
machine-learning neural-network deep-learning lstm rnn
New contributor
Zubera is a new contributor to this site. Take care in asking for clarification, commenting, and answering.
Check out our Code of Conduct.
$endgroup$
On a time series problem that we try to solve using RNNs
, the input usually has the shape $input features times timesteps times batchsize$ and we then feed this input into recurrent layers. An alternative would be to flatten the data so that the shape is $(input features times timesteps) times batchsize$ and use a fully connected layer for our time series task. This would clearly work and our dense network
would be able to find dependencies between the data at different timesteps as well. So what is it that makes recurrent layers more powerful? I would be very thankful for an intuitive explanation.
machine-learning neural-network deep-learning lstm rnn
machine-learning neural-network deep-learning lstm rnn
New contributor
Zubera is a new contributor to this site. Take care in asking for clarification, commenting, and answering.
Check out our Code of Conduct.
New contributor
Zubera is a new contributor to this site. Take care in asking for clarification, commenting, and answering.
Check out our Code of Conduct.
edited 5 mins ago


Media
7,07562061
7,07562061
New contributor
Zubera is a new contributor to this site. Take care in asking for clarification, commenting, and answering.
Check out our Code of Conduct.
asked 2 hours ago
ZuberaZubera
61
61
New contributor
Zubera is a new contributor to this site. Take care in asking for clarification, commenting, and answering.
Check out our Code of Conduct.
New contributor
Zubera is a new contributor to this site. Take care in asking for clarification, commenting, and answering.
Check out our Code of Conduct.
Zubera is a new contributor to this site. Take care in asking for clarification, commenting, and answering.
Check out our Code of Conduct.
add a comment |
add a comment |
1 Answer
1
active
oldest
votes
$begingroup$
The first reason is the number of parameters. The former case that you've mentioned, for each neuron there should be corresponding entries that would increase the number of training parameters. The other reason is that by employing simple feed-forward neurons you are somehow discarding the temporal information of your data which means you are discarding the sequence information in your data. This is somehow like the spatial data which is obtained by convolutional layers in CNNs
.
$endgroup$
add a comment |
Your Answer
StackExchange.ifUsing("editor", function () {
return StackExchange.using("mathjaxEditing", function () {
StackExchange.MarkdownEditor.creationCallbacks.add(function (editor, postfix) {
StackExchange.mathjaxEditing.prepareWmdForMathJax(editor, postfix, [["$", "$"], ["\\(","\\)"]]);
});
});
}, "mathjax-editing");
StackExchange.ready(function() {
var channelOptions = {
tags: "".split(" "),
id: "557"
};
initTagRenderer("".split(" "), "".split(" "), channelOptions);
StackExchange.using("externalEditor", function() {
// Have to fire editor after snippets, if snippets enabled
if (StackExchange.settings.snippets.snippetsEnabled) {
StackExchange.using("snippets", function() {
createEditor();
});
}
else {
createEditor();
}
});
function createEditor() {
StackExchange.prepareEditor({
heartbeatType: 'answer',
autoActivateHeartbeat: false,
convertImagesToLinks: false,
noModals: true,
showLowRepImageUploadWarning: true,
reputationToPostImages: null,
bindNavPrevention: true,
postfix: "",
imageUploader: {
brandingHtml: "Powered by u003ca class="icon-imgur-white" href="https://imgur.com/"u003eu003c/au003e",
contentPolicyHtml: "User contributions licensed under u003ca href="https://creativecommons.org/licenses/by-sa/3.0/"u003ecc by-sa 3.0 with attribution requiredu003c/au003e u003ca href="https://stackoverflow.com/legal/content-policy"u003e(content policy)u003c/au003e",
allowUrls: true
},
onDemand: true,
discardSelector: ".discard-answer"
,immediatelyShowMarkdownHelp:true
});
}
});
Zubera is a new contributor. Be nice, and check out our Code of Conduct.
Sign up or log in
StackExchange.ready(function () {
StackExchange.helpers.onClickDraftSave('#login-link');
});
Sign up using Google
Sign up using Facebook
Sign up using Email and Password
Post as a guest
Required, but never shown
StackExchange.ready(
function () {
StackExchange.openid.initPostLogin('.new-post-login', 'https%3a%2f%2fdatascience.stackexchange.com%2fquestions%2f46559%2fwhy-do-recurrent-layers-work-better-than-simple-feed-forward-networks%23new-answer', 'question_page');
}
);
Post as a guest
Required, but never shown
1 Answer
1
active
oldest
votes
1 Answer
1
active
oldest
votes
active
oldest
votes
active
oldest
votes
$begingroup$
The first reason is the number of parameters. The former case that you've mentioned, for each neuron there should be corresponding entries that would increase the number of training parameters. The other reason is that by employing simple feed-forward neurons you are somehow discarding the temporal information of your data which means you are discarding the sequence information in your data. This is somehow like the spatial data which is obtained by convolutional layers in CNNs
.
$endgroup$
add a comment |
$begingroup$
The first reason is the number of parameters. The former case that you've mentioned, for each neuron there should be corresponding entries that would increase the number of training parameters. The other reason is that by employing simple feed-forward neurons you are somehow discarding the temporal information of your data which means you are discarding the sequence information in your data. This is somehow like the spatial data which is obtained by convolutional layers in CNNs
.
$endgroup$
add a comment |
$begingroup$
The first reason is the number of parameters. The former case that you've mentioned, for each neuron there should be corresponding entries that would increase the number of training parameters. The other reason is that by employing simple feed-forward neurons you are somehow discarding the temporal information of your data which means you are discarding the sequence information in your data. This is somehow like the spatial data which is obtained by convolutional layers in CNNs
.
$endgroup$
The first reason is the number of parameters. The former case that you've mentioned, for each neuron there should be corresponding entries that would increase the number of training parameters. The other reason is that by employing simple feed-forward neurons you are somehow discarding the temporal information of your data which means you are discarding the sequence information in your data. This is somehow like the spatial data which is obtained by convolutional layers in CNNs
.
answered 7 mins ago


MediaMedia
7,07562061
7,07562061
add a comment |
add a comment |
Zubera is a new contributor. Be nice, and check out our Code of Conduct.
Zubera is a new contributor. Be nice, and check out our Code of Conduct.
Zubera is a new contributor. Be nice, and check out our Code of Conduct.
Zubera is a new contributor. Be nice, and check out our Code of Conduct.
Thanks for contributing an answer to Data Science Stack Exchange!
- Please be sure to answer the question. Provide details and share your research!
But avoid …
- Asking for help, clarification, or responding to other answers.
- Making statements based on opinion; back them up with references or personal experience.
Use MathJax to format equations. MathJax reference.
To learn more, see our tips on writing great answers.
Sign up or log in
StackExchange.ready(function () {
StackExchange.helpers.onClickDraftSave('#login-link');
});
Sign up using Google
Sign up using Facebook
Sign up using Email and Password
Post as a guest
Required, but never shown
StackExchange.ready(
function () {
StackExchange.openid.initPostLogin('.new-post-login', 'https%3a%2f%2fdatascience.stackexchange.com%2fquestions%2f46559%2fwhy-do-recurrent-layers-work-better-than-simple-feed-forward-networks%23new-answer', 'question_page');
}
);
Post as a guest
Required, but never shown
Sign up or log in
StackExchange.ready(function () {
StackExchange.helpers.onClickDraftSave('#login-link');
});
Sign up using Google
Sign up using Facebook
Sign up using Email and Password
Post as a guest
Required, but never shown
Sign up or log in
StackExchange.ready(function () {
StackExchange.helpers.onClickDraftSave('#login-link');
});
Sign up using Google
Sign up using Facebook
Sign up using Email and Password
Post as a guest
Required, but never shown
Sign up or log in
StackExchange.ready(function () {
StackExchange.helpers.onClickDraftSave('#login-link');
});
Sign up using Google
Sign up using Facebook
Sign up using Email and Password
Sign up using Google
Sign up using Facebook
Sign up using Email and Password
Post as a guest
Required, but never shown
Required, but never shown
Required, but never shown
Required, but never shown
Required, but never shown
Required, but never shown
Required, but never shown
Required, but never shown
Required, but never shown
RuI9j,nEneDgidBn0AKpaw,5RA96f lYrS3DzTN9T,zBtJJ7M5i